Essential Insights on "Hands-On Machine Learning" Book Review
Written on
Chapter 1: Overview of the Book
This review delves into the book "Hands-On Machine Learning with Scikit-learn, TensorFlow & Keras," a prominent resource in the field of machine learning. Having recently completed the book, I felt compelled to share my thoughts, as it has proven to be an invaluable asset for both newcomers and seasoned practitioners in machine learning. Published by O'Reilly, this book is characterized by its exceptional content quality and is crafted specifically for those starting their journey in machine learning. It encompasses a wide array of topics, including theoretical foundations, practical coding exercises, and self-assessment questions. Notably, the book excels in delivering clear explanations alongside relevant code samples.
The Book's Comprehensive Content
One standout feature of this book is its extensive coverage of machine learning concepts. For a detailed overview of the content, you can check it out here; it's quite substantial! However, based on my personal experience, you need not read every section in one sitting. I found it more beneficial to focus on the chapters pertinent to my ongoing machine learning projects, which extended over a period of 1–2 years. This duration wasn't due to a lack of diligence on my part; rather, it was the result of my commitment to exploring various machine learning domains, including text processing, image analysis, supervised learning, unsupervised learning, and reinforcement learning.
One of the primary advantages of having this book is the ability to revisit chapters as needed, using them as a reference while learning. For example, during my first text-oriented Kaggle competition, I faced challenges with RNNs and LSTMs, which prompted me to revisit those specific chapters multiple times. It was reassuring to have a consolidated source of high-quality content, complete with code examples, all in one location.
Practical Aspects of Learning
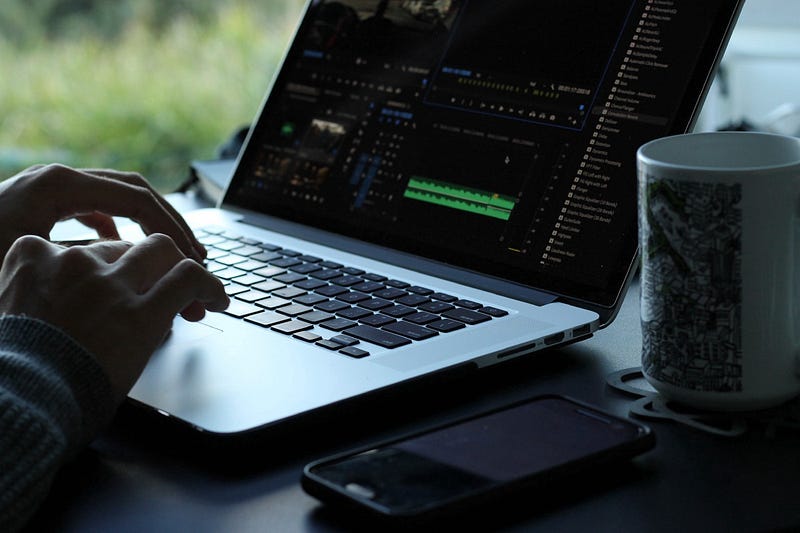
Photo by Matthew Kwong on Unsplash
I tend to prefer hands-on problem-solving over repetitive reading, especially in programming, as I believe that practical experience is invaluable. However, with machine learning, I discovered that a solid theoretical foundation is essential for understanding each algorithm and model. Striking a balance between in-depth exploration and practical application is crucial for becoming a proficient ML engineer or data scientist. If one delves too deeply into a single model, they risk narrowing their focus; conversely, a superficial understanding across many models can hinder expertise.
In my view, this book achieves an admirable equilibrium between detailed learning and practical coding, which has significantly enriched my ongoing machine learning education.
Should You Consider Purchasing It?
Unless you have already spent 1–2 years immersed in machine learning, I believe this book will offer tremendous value. There are numerous aspects of ML that you might not yet have encountered, and this book can illuminate those areas for your upcoming projects. Additionally, it features several general chapters on constructing ML projects and pipelines from the ground up—insights I found particularly beneficial. While it's tempting to dive deep into the minutiae of various models, occasionally stepping back to view the entire process holistically can be incredibly beneficial.
Another aspect I appreciated is the book's relevance; the examples and models discussed are quite modern and are likely to remain applicable for some time to come. I found it especially useful during lengthy projects, recalling how I often revisited specific chapters during my undergraduate thesis.
Final Thoughts
I hope this brief review has provided useful insights. If you have differing opinions about the book, I would love to hear your thoughts in the comments section below.
You can purchase the book here. For more explanations on software engineering concepts, tutorials, and updates, subscribe with your email!
This first video review highlights essential takeaways and insights from "Hands-On Machine Learning with Scikit-Learn, Keras, & TensorFlow."
The second video explores whether "Hands-On Machine Learning" is the best book available on the subject, providing an in-depth review.