The Evolution of Reinforcement Learning: A Journey Through Time
Written on
Chapter 1: The Origins of Reinforcement Learning
The story of Reinforcement Learning (RL) is quite fascinating, beginning not in the realm of technology but rather in psychology. It's interesting to note that RL's historical journey has its roots in the behavioral studies of animals.
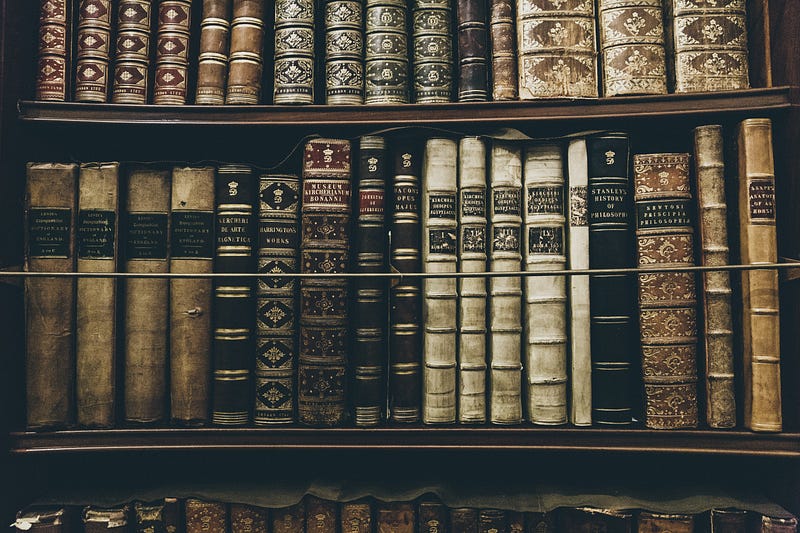
Photo by Thomas Kelley on Unsplash
In the 1930s, behavioral psychologist B. F. Skinner embarked on research that would shape the understanding of learning through positive reinforcement. His experiments with pigeons and rats illustrated how animals could be trained to execute complex tasks simply by providing rewards, such as food, for desired behaviors. This foundational work highlighted that both animals and agents could adapt their actions based on previous experiences, a concept that is central to the principles of RL.
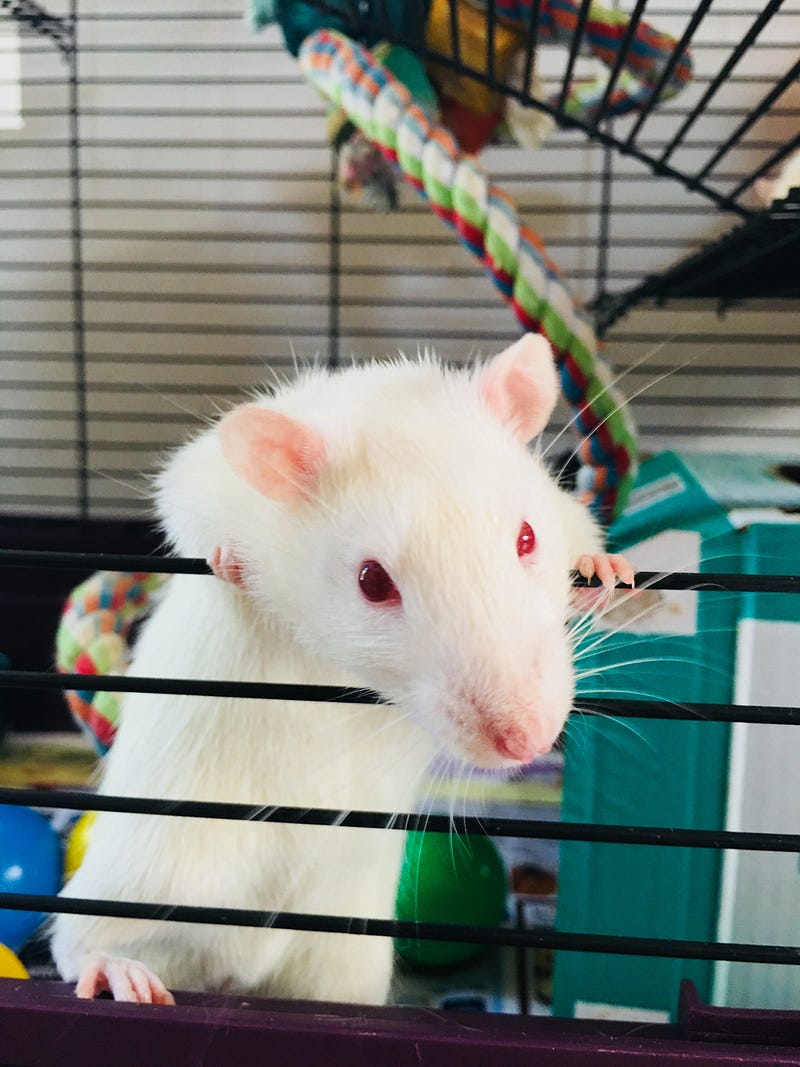
Photo by Annemarie Horne on Unsplash
Today, RL is recognized as a branch of machine learning, where computers learn to enhance their performance on specific tasks through rewards and punishments. Despite being a relatively recent development in machine learning, its roots can be traced back to the foundational theories of animal behavior and operant conditioning established by Skinner.
Chapter 2: Transition to Computational Models
The shift from psychological theories to computational frameworks began in the 1950s and 1960s. During this period, researchers started to apply RL techniques to artificial intelligence (AI) tasks.
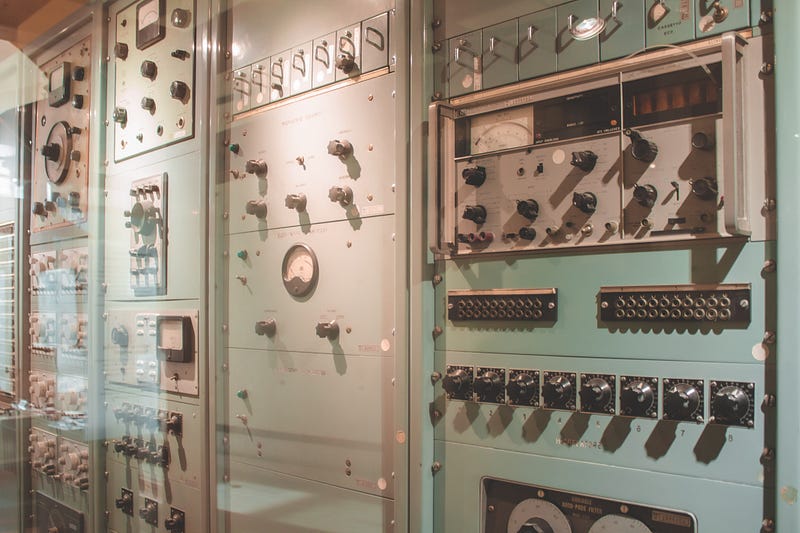
Photo by Diane Picchiottino on Unsplash
Richard Bellman was a key figure in this evolution, introducing dynamic programming concepts that would eventually lead to the formulation of the Bellman Equations. These equations play a critical role in RL, allowing agents to derive optimal policies once resolved. This means that an RL agent can learn to navigate various environments—be it a maze, a race track, or the stock market—by utilizing these foundational equations.
The field continued to evolve with contributions from researchers like Samuel and Sutton, gaining wider acceptance in the late 1980s and early 1990s. It was during this time that influential papers began to emerge, further solidifying RL's importance in both academia and practical applications.
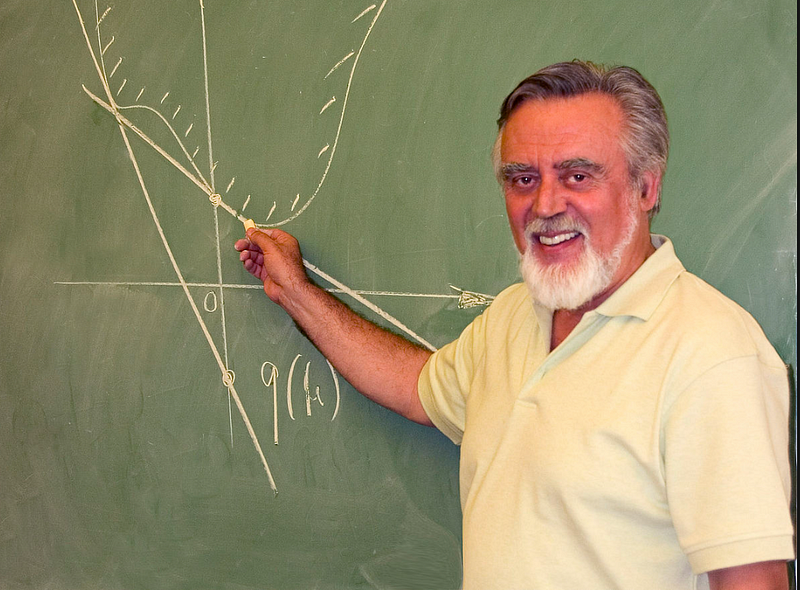
Chapter 3: Modern Contributions and Future Directions
The landscape of RL has been shaped by remarkable figures such as Dimitri Bertsekas and Richard Sutton. Bertsekas, known for his work in dynamic programming, has inspired many with his textbooks on optimal control. Meanwhile, Sutton has significantly influenced the field through his teaching and publications, making his textbook an essential resource for those new to RL.
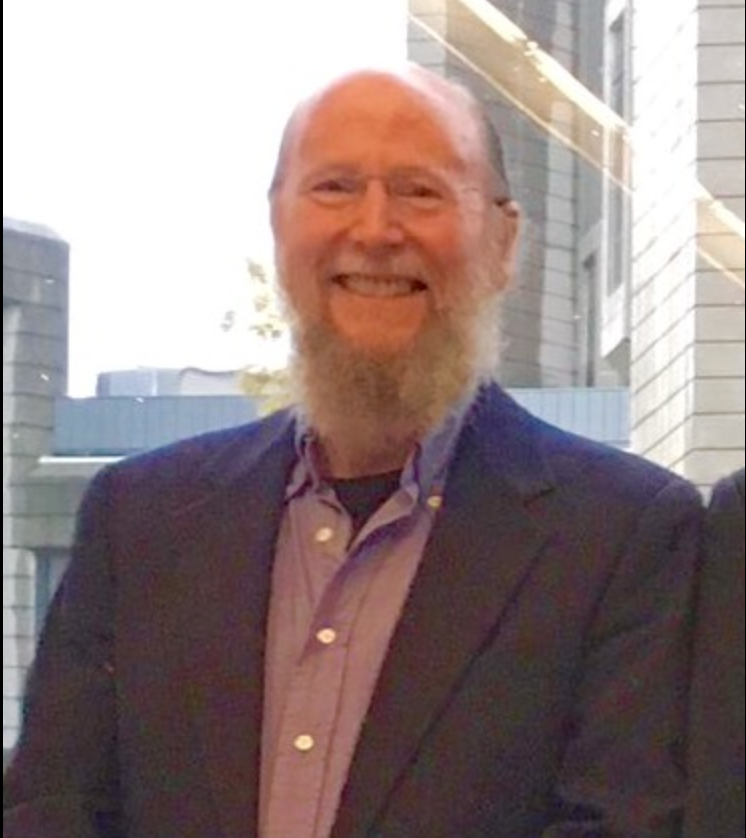
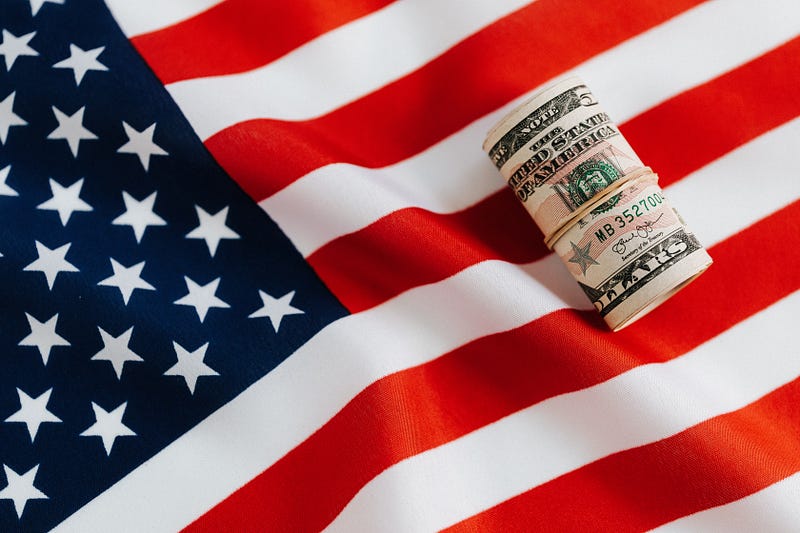
Photo by Karolina Grabowska on Pexels
As RL continues to advance, its applications expand across numerous fields, demonstrating its potential to address some of society's most pressing challenges. The integration of deep learning has propelled RL to new heights, achieving remarkable results across various tasks. Looking ahead, RL is poised to remain a crucial element in both AI research and its applications across industries.
In this video titled "A History of Reinforcement Learning," Prof. A.G. Barto explores the foundational concepts and milestones that have shaped RL into what it is today.
The second video, "RL Course by David Silver - Lecture 1: Introduction to Reinforcement Learning," provides an introduction to RL concepts and their significance in the AI landscape.
Until next time,
Caleb.